Redirecting to the OSCA book...
Maintainers should update their links to point to the new location.
Wait a moment, we are redirecting you now:
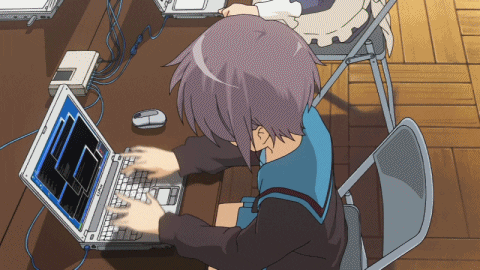
Maintainers should update their links to point to the new location.
Wait a moment, we are redirecting you now: